AI has revolutionized the way IT security professionals think about cybersecurity. Newer AI-powered cybersecurity tools and systems have the ability to support providing even better data protection against threats by quickly recognizing behavior patterns, automating processes, and detecting anomalies.
AI in Cybersecurity
AI powered cybersecurity can monitor, analyze detect, and respond to cyber threats in real time. As AI algorithms analyze massive amounts of data to detect patterns that are indicative of a cyber threat, it can also scan the entire network for weaknesses to prevent common kinds of cyber attacks.
AI primarily monitors and analyzes behavior patterns. Using these patterns to create a baseline, AI can detect unusual behaviors and restrict unauthorized access to systems. AI can also help to prioritize risk, instantly detect the possibility of malware and intrusions before they begin.
When implemented properly, AI can serve as the engine for security automation, which frees up the time and resources of employees by automating repetitive tasks. AI can also reduce the occurrence of human error by removing humans from a task or process.
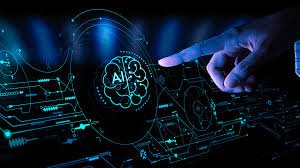
How Is AI Cybersecurity Different?
Cybersecurity protection with artificial intelligence will never fully replace security professionals, as there will always be a need for creative problem-solving and more complex challenges in the workplace. However, AI can and already does assist human security professionals by analyzing vast amounts of data, recognizing patterns, and creating insights based on large volumes of security data. This could take hours, sometimes weeks to complete with traditional security processes.
Before AI, security professionals used signature-based detection tools and systems to identify potential cyber threats. These security tools compare incoming network traffic to a database of known threats or malicious code signatures. Upon detection, the system triggers an alert and suggests to the security professional that they should take an action to block or quarantine the threat.
This signature-based security approach has been reasonably effective against known threats. However, the signature-based detection approach has proven to be inadequate against new (Zero-Day) or unknown threats. Too often, these tools also resulted in a higher frequency of false positives, which sent security professionals on a “wild goose chase.”
Traditional cybersecurity also relies heavily on manual analysis. Security analysts must manually investigate security alerts and event logs in search of any identifiable patterns that serve as indicators of a potential security breach. Investigating logs and events can take extensive amounts of time and relying solely on a single security analyst is a mistake companies cannot afford to make.
Advantage of AI in Cybersecurity
1. Threat Detection and Prevention
AI-powered systems excel in real-time threat detection, swiftly identifying and neutralizing potential risks. Through sophisticated algorithms, these systems leverage behavioral analysis and anomaly detection to spot and halt evolving threats before they infiltrate networks or systems.
2. Real-time Threat Detection
AI algorithms continuously monitor network traffic, instantly recognizing suspicious patterns or deviations from normal behavior, enabling rapid response to potential breaches.
3. Advanced Malware Detection
AI-driven tools possess the capability to recognize and counteract even the most complex malware strains by analyzing their signatures and behaviors, bolstering defense against evolving cyber threats.
4. Automation and Efficiency
One of AI’s prominent advantages is its ability to automate mundane security tasks, significantly enhancing efficiency and allowing human experts to focus on more complex security challenges.
5. Streamlining Security Operations
AI streamlines and optimizes routine tasks like log analysis and system monitoring, freeing up cybersecurity professionals to concentrate on strategic initiatives.
6. Automated Response to Attacks
These systems can autonomously respond to threats by implementing predefined response protocols, reducing response times and minimizing potential damages.
7. Adaptability and Learning
Machine learning models continually evolve and adapt to new threats and attack vectors, constantly learning from previous encounters to fortify defenses.
8. Machine Learning for Pattern Recognition
AI models employ machine learning algorithms to identify patterns in historical data, aiding in the identification of new threats based on similarities to previous attacks.
9. Continuous Improvement in Security
The iterative nature of AI-based systems ensures ongoing improvements in threat detection and response, staying ahead of emerging risks.
10. Handling Vast Amounts of Data
AI excels in processing and analyzing massive volumes of data, a capability crucial in today’s data-driven cybersecurity landscape.
11. Big Data Analysis for Security Insights
AI systems utilize big data analytics to derive actionable insights from extensive datasets, empowering security teams with valuable information for preemptive measures.
12. Scalability in Security Measures
These systems offer scalable solutions, accommodating the exponential growth of data and potential threats without compromising efficiency.
Disadvantage of AI in Cybersecurity
1. Bias and Errors
Despite their sophistication, AI algorithms can be susceptible to biases inherited from training data, leading to erroneous decisions and potentially overlooking certain threats.
2. Ethical Implications in AI Decision-Making
The ethical implications of biased AI decision-making raise concerns regarding fairness and accuracy, demanding constant scrutiny and mitigation efforts.
3. Challenges in Algorithmic Accuracy
AI systems might occasionally misinterpret benign activities as threats or fail to recognize new attack patterns due to limitations in their algorithms.
4. Sophisticated Attacks on AI
Cybercriminals are targeting AI systems, aiming to manipulate or exploit vulnerabilities within these systems to evade detection or launch sophisticated attacks.
5. Threats Targeting AI Systems
Malicious actors are devising methods to manipulate AI algorithms through adversarial attacks, aiming to deceive AI-based security measures.
6. Security Risks in AI Models
Flaws in AI models or vulnerabilities in their implementation pose risks, potentially leading to exploitation by cyber attackers.
7. Dependency and Overreliance
Overreliance on AI-driven security measures might lead to complacency, potentially diminishing the role of human expertise in cybersecurity.
8. Potential Human Skill Erosion
Heavy reliance on AI could diminish the critical thinking and problem-solving skills of cybersecurity professionals, affecting their ability to handle unforeseen threats.
10. Vulnerabilities in AI-Centric Systems
Complete dependence on AI systems might create a single point of failure, leaving organizations vulnerable if these systems are compromised.
11. Complexity and Implementation Challenges
The integration of AI into existing cybersecurity infrastructure can pose implementation challenges, including compatibility issues and a shortage of skilled personnel.
12. Integration Issues in Existing Systems
Harmonizing AI systems with legacy security infrastructure might pose compatibility challenges, requiring meticulous planning and execution.
13. Skill Gap in AI Implementation and Management
The shortage of skilled professionals proficient in both cybersecurity and AI presents a hurdle in effectively deploying and managing AI-based security systems.
What Are the Risks of AI in Cybersecurity?
Like any technology, AI can be used for good or malicious purposes. Threat actors can use some of the same AI tools designed to help humanity to commit fraud, scams, and other cybercrimes.
1: Cyber attacks optimization
Experts say that attackers can use generative AI and large language models to scale attacks at an unseen level of speed and complexity. They may use generative AI to find fresh ways to undermine cloud complexity and take advantage of geopolitical tensions for advanced attacks. They can also optimize their ransomware and phishing attack techniques by polishing them with generative AI.
2: Automated malware
An AI like ChatGPT is excellent at accurately crunching numbers. According to Columbia Business School professor Oded Netzer, ChatGPT can already “write code quite well.”
While software like ChatGPT has some protections to prevent users from creating malicious code, experts can use clever techniques to bypass it and create malware. For example, one researcher was able to find a loophole and create a nearly undetectable complex data-theft executable. The executable had the sophistication of malware created by a state-sponsored threat actor*.
This could be the tip of the iceberg. Future AI-powered tools may allow developers with entry-level programming skills to create automated malware, like an advanced malicious bot. A malicious bot can steal data, infect networks, and attack systems with little to no human intervention.
3: Physical safety
As more systems such as autonomous vehicles, manufacturing and construction equipment, and medical systems use AI, risks of artificial intelligence to physical safety can increase. For example, an AI-based true self-driving car that suffers a cyber security breach could result in risks to the physical safety of its passengers. Similarly, the dataset for maintenance tools at a construction site could be manipulated by an attacker into creating hazardous conditions.
AI privacy risks
In what was an embarrassing bug for OpenAI CEO Sam Altman,ChatGPT leaked bits of chat history of other users. Although the bug was fixed, there are other possible privacy risks due to the vast amount of data that AI crunches. For example, a hacker who breaches an AI system could access different kinds of sensitive information.
An AI system designed for marketing, advertising, profiling, or surveillance could also threaten privacy in ways George Orwell couldn’t fathom. In some countries, AI-profiling technology is already helping states invade user privacy.
Stealing AI models
There are some risks of AI model theft through network attacks, social engineering techniques, and vulnerability exploitation by threat actors such as state-sponsored agents, insider threats like corporate spies, and run-of-the-mill computer hackers. Stolen models can be manipulated and modified to assist attackers with different malicious activities, compounding artificial intelligence risks to society. AI privacy risks
In what was an embarrassing bug for OpenAI CEO Sam Altman,ChatGPT leaked bits of chat history of other users. Although the bug was fixed, there are other possible privacy risks due to the vast amount of data that AI crunches. For example, a hacker who breaches an AI system could access different kinds of sensitive information.
An AI system designed for marketing, advertising, profiling, or surveillance could also threaten privacy in ways George Orwell couldn’t fathom. In some countries, AI-profiling technology is already helping states invade user privacy.
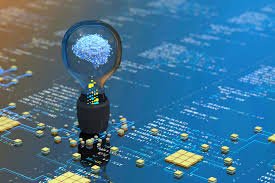
Why is AI in cybersecurity important?
. Enhanced Threat Detection: AI systems can analyze vast amounts of data quickly to identify anomalies and potential threats that might be missed by traditional methods. This capability is crucial for identifying sophisticated and emerging threats in real time.
2. Improved Response Time: AI can automate responses to certain types of cyber incidents, reducing the time between detection and mitigation. This rapid response helps limit the damage caused by cyberattacks.
3. Proactive Defense: AI enables predictive analytics, allowing organizations to anticipate and prepare for potential cyber threats before they occur. This proactive approach enhances overall security posture.
4. Handling Complexity: Modern cyber threats are increasingly complex and can involve multiple attack vectors. AI can integrate and analyze diverse data sources, providing a comprehensive view of the threat landscape and enabling more effective defense strategies.
5. Reducing Workload for Security Teams: By automating routine and time-consuming tasks such as threat hunting, monitoring, and incident response, AI allows cybersecurity professionals to focus on more strategic activities, improving overall efficiency and effectiveness.
6. Scalability: AI solutions can scale to handle large volumes of data and an increasing number of devices connected to networks, maintaining robust security in the face of growing digital infrastructure.
What is Machine Learning (ML)?
Machine learning (ML) is a branch of artificial intelligence (AI) and computer science that focuses on the using data and algorithms to enable AI to imitate the way that humans learn, gradually improving its accuracy.

How does machine learning work?
Machine learning is a subset of artificial intelligence that allows computers to learn from data without being explicitly programmed.
Data Collection: Gathering relevant data is the first step. This data can be anything from images, text, numbers, or a combination.
Data Preparation: The collected data is cleaned, organized, and transformed into a suitable format for the machine learning model.
Choosing an Algorithm: Selecting the right algorithm depends on the problem you’re trying to solve.
- Supervised Learning: The algorithm learns from labeled data (data with correct answers). Examples: classification (spam detection) and regression (predicting house prices).
- Unsupervised Learning: The algorithm finds patterns in unlabeled data. Examples: clustering (grouping similar data points) and association rule learning (finding relationships between items).
- Reinforcement Learning: The algorithm learns by interacting with an environment and receiving rewards or penalties. Examples: game playing, robotics.
Training the Model: The algorithm processes the prepared data to learn patterns and relationships. This process is called training.
Evaluation: The model’s performance is assessed on a separate dataset to see how well it generalizes to new data.
Prediction or Decision Making: Once trained and evaluated, the model can be used to make predictions or decisions on new data.
Machine learning methods
Supervised machine learning
Supervised learning, also known as supervised machine learning, is defined by its use of labeled datasets to train algorithms to classify data or predict outcomes accurately. As input data is fed into the model, the model adjusts its weights until it has been fitted appropriately. This occurs as part of the cross validation process to ensure that the model avoids overfitting or underfitting. Supervised learning helps organizations solve a variety of real-world problems at scale, such as classifying spam in a separate folder from your inbox. Some methods used in supervised learning include neural networks, naïve bayes, linear regression, logistic regression, random forest, and support vector machine (SVM).
Unsupervised machine learning
Unsupervised learning, also known as unsupervised machine learning, uses machine learning algorithms to analyze and cluster unlabeled datasets (subsets called clusters). These algorithms discover hidden patterns or data groupings without the need for human intervention. This method’s ability to discover similarities and differences in information make it ideal for exploratory data analysis, cross-selling strategies, customer segmentation, and image and pattern recognition. It’s also used to reduce the number of features in a model through the process of dimensionality reduction. Principal component analysis (PCA) and singular value decomposition (SVD) are two common approaches for this. Other algorithms used in unsupervised learning include neural networks, k-means clustering, and probabilistic clustering methods.
Semi-supervised learning
Semi-supervised learning offers a happy medium between supervised and unsupervised learning. During training, it uses a smaller labeled data set to guide classification and feature extraction from a larger, unlabeled data set. Semi-supervised learning can solve the problem of not having enough labeled data for a supervised learning algorithm. It also helps if it’s too costly to label enough data.
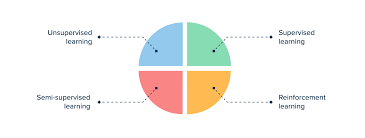
Tools for Cybersecurity
1. Darktrace
Darktrace is a good AI tools for cybersecurity that helps in identifying any ongoing threat. It is highly used in forensic investigations. It highly utilizes machine learning and becomes the immune system and a great defense mechanism. It is considered as one of the best AI tools for Cybersecurity.
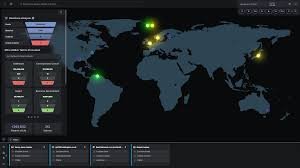
2. Cylance
Cylance is an AI tools for cybersecurity that is a part of BlackBerry and it highly focuses on giving pre-directory solutions with endpoint security solutions. Threat hunting and acting as a lightweight agent is the best for protection.

3. Vectra AI
Vectra AI is highly focused on detecting threats and leverages other AI tools for cybersecurity as has unique algorithms to respond to high-quality threats as well. It has continuous real-time monitoring of network traffic, user activity, and cloud environments enabling enterprises to discover and mitigate risks more quickly.
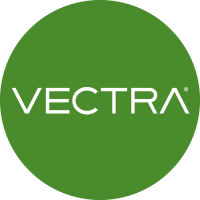
4. SentinelOne
This AI tools for cybersecurity platform offers security teams and threat-hunting capabilities. It allows the security team to investigate the actual problem involved. It also analyses the endpoint threat with comprehensive visibility.

Conclusion
Artificial Intelligence (AI) is undeniably revolutionizing the landscape of cybersecurity. Its ability to process vast amounts of data, identify patterns, and learn from experience offers unprecedented advantages in threat detection, prevention, and response. AI-powered systems can outpace human analysts in identifying zero-day vulnerabilities, detecting anomalies, and predicting potential attacks.
However, the same technology that empowers defenders can also be weaponized by attackers. AI-driven cyberattacks are becoming increasingly sophisticated, capable of adapting to defensive measures and launching highly targeted campaigns. This creates a dynamic and complex arms race between defenders and attackers.
To fully realize the potential of AI in cybersecurity, a balanced approach is essential. Organizations must invest in AI-driven solutions while simultaneously implementing robust safeguards to protect against AI-powered threats. Ethical considerations and responsible AI development are paramount to mitigate risks and ensure that AI is used for good.
Key takeaways:
- AI is a powerful tool for enhancing cybersecurity: It can significantly improve threat detection, prevention, and response capabilities.
- AI poses new challenges: Attackers can leverage AI to create more sophisticated and evasive threats.
- A balanced approach is crucial: Organizations must invest in AI-driven defenses while addressing potential risks.
- Ethical considerations are essential: Responsible AI development is vital for mitigating risks and ensuring AI is used for good.
The future of cybersecurity will be defined by the interplay between AI and human expertise. By harnessing the power of AI while maintaining a strong human element, organizations can build a more resilient and secure digital landscape.
FAQs
AI can correlate data from various sources, providing a holistic view of security events and aiding in the identification of complex attack patterns or insider threat behavior. It also helps prioritize threats by assessing their severity, ensuring that security teams can address the most critical risks to data first.
Future of Artificial Intelligence. Artificial intelligence (AI) has a bright future, but it also faces several difficulties. AI is predicted to grow increasingly pervasive as technology develops, revolutionising sectors including healthcare, banking, and transportation.
Within the world of cyber security, AI is filling—or assisting with—a number of roles and processes. It’s being used to analyze logs, predict threats, read source code, identify vulnerabilities and even to create or exploit vulnerabilities.